PyPy and conda-forge
You can use PyPy as your python interpreter in a conda environment. The conda-forge team has graciously provided this service.
The conda-forge tips-and-tricks page says:
The conda-forge channel supports creating and installing packages into environments using the PyPy interpreter. Many packages are already available. You need to enable the conda-forge channel and use the pypy identifier when creating your environment:
$ conda create -c conda-forge -n my-pypy-env pypy python=3.8 $ conda activate my-pypy-env
Currently supported python versions are 3.8 and 3.9. Support for pypy3.7 has been dropped. While you can still create a python 3.7 environment, you you will not be getting updates as new package versions are released (including pypy itself).
if you are using defaults as a low priority channel, then you need to use strict channel priority as the metadata in defaults has not been patched yet which allows cpython extension packages to be installed alongside pypy.
$ conda config --set channel_priority strict
The work required some out-of-the-box thinking on the part of conda-forge since
they needed to add the idea of a pypy
identifier to the python version and
the whole conda team has been very supportive of the effort needed. Binary
packages are on offer for the usual platforms:
-
x86_64
windows, macos, linux -
ppc64le
andaarch64
linux.
There are currently over 1000 packages available for download via the conda-forge channel, and more are being added as the kind package maintainers work around various differences between CPython and PyPy. Please let us know if your favorite package is not supported.
The PyPy Blog Turns 15 Years
Exactly 15 years ago today we wrote the first blog post on the PyPy blog! Over the years, we have written 423 posts, from the shortest to the longest. In 2021 we moved from blogger to our own domain.
The topics over the years varied widely, we published release announcements; roadmaps; JIT, GC and STM updates; benchmarks; sprint, trip and conference reports; technical deep dives; case studies; april fool's jokes; research projects; other languages using RPython; finished PhD Bachelor and Master, theses; pictures:
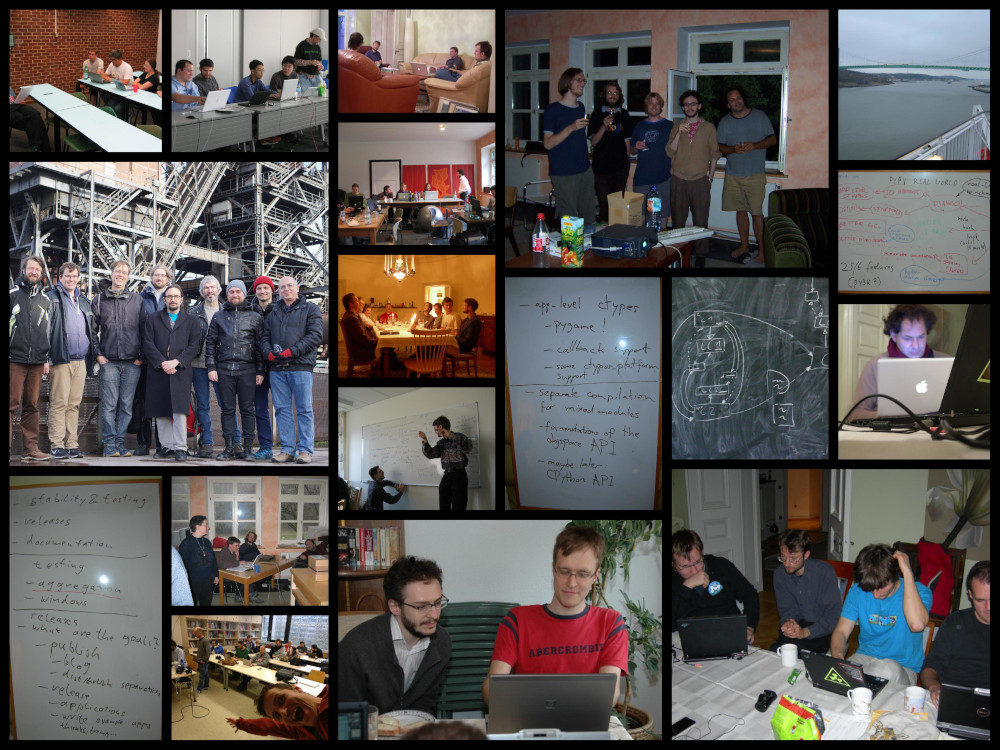
and diagrams:
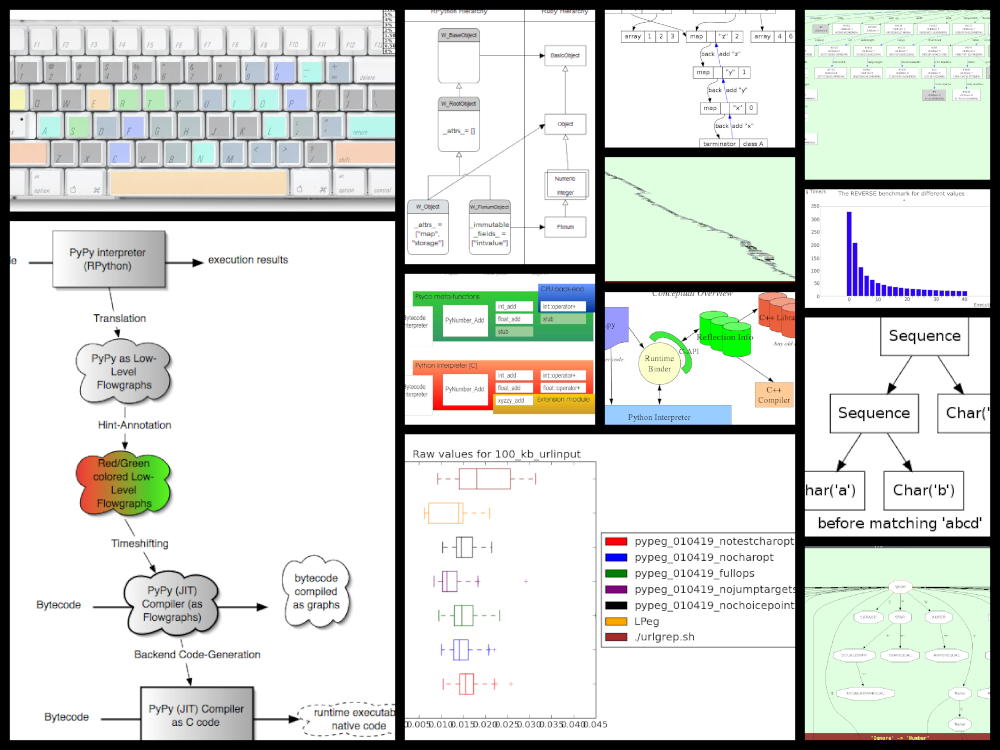
Quite a number of blog posts were very early iterations of papers that we published later, here are a few that I can remember:
Applying a Tracing JIT to an Interpreter became Tracing the meta-level: PyPy's tracing JIT compiler at ICOOOLPS 2009, by far our most successful paper.
Escape Analysis in PyPy's JIT became Allocation removal by partial evaluation in a tracing JIT at PEPM 2010.
Controlling the Tracing of an Interpreter With Hints was a draft of the paper Runtime feedback in a meta-tracing JIT for efficient dynamic languages at ICOOOLPS 2011
Using Escape Analysis Across Loop Boundaries for Specialization was the nucleus of Loop-aware optimizations in PyPy's tracing JIT at DLS 2012.
List Strategies was eventually turned into the paper Storage strategies for collections in dynamically typed languages at OOPSLA 2013.
Greatest Hits
In terms of visitors, the top five posts on the old blog were – on the new blog we simply don't have stats (yet?):
The number of posts per year developed like this:
The most prolific authors are:
Several blog posts have made it to the Hacker News front page, three of them to number 1:
Personal Favourites
While looking through the posts, there were a few that stood out to me in some way, so here's a subjective list of ones that I had fun looking at again:
2009: PyPy gets a new compiler
2012: Architecture of Cppyy
2013: 10 years of PyPy
2014: PyPy IO Improvements
2016: PyPy Enterprise Edition
We'd like to thank our authors, guest authors, commenters, users and readers who have stuck with us through one and a half decades! If there's any particular topics you would like to read something about, or any guest posts you'd like to write, let us know!
Allocation Removal in the Toy Optimizer
One of the workhorse optimization of RPython's tracing JIT is allocation removal, which removes short-lived object allocation from traces. Many Python programs create a lot of objects that only live for a short time, and whose lifespan is fully predictable (common examples are integer and float boxes, but also tuples, frames, intermediate string results, etc). Allocation removal will try (and very often succeed) to remove these allocations from traces. In this blog post I want to show a toy version of how allocation removal is implemented.
In the previous blog post of this series I showed the complete code for writing a toy one-pass optimizer that does constant folding, common subexpression elimination and strength reduction. In this second post, I want to use allocation removal as a more advanced optimization pass. The basic optimization framework is the same, we will use the same datastructures for intermediate representation and also keep using the same union find data structure to store equivalences between IR operations. Here's the infrastructure code from the last post:
import pytest from typing import Optional, Any class Value: def find(self): raise NotImplementedError("abstract") def _set_forwarded(self, value): raise NotImplementedError("abstract") class Operation(Value): def __init__( self, name: str, args: list[Value] ): self.name = name self.args = args self.forwarded = None self.info = None def __repr__(self): return ( f"Operation({self.name}, " f"{self.args}, {self.forwarded}, " f"{self.info})" ) def find(self) -> Value: op = self while isinstance(op, Operation): next = op.forwarded if next is None: return op op = next return op def arg(self, index): return self.args[index].find() def make_equal_to(self, value: Value): self.find()._set_forwarded(value) def _set_forwarded(self, value: Value): self.forwarded = value class Constant(Value): def __init__(self, value: Any): self.value = value def __repr__(self): return f"Constant({self.value})" def find(self): return self def _set_forwarded(self, value: Value): assert ( isinstance(value, Constant) and value.value == self.value ) class Block(list): def opbuilder(opname): def wraparg(arg): if not isinstance(arg, Value): arg = Constant(arg) return arg def build(self, *args): # construct an Operation, wrap the # arguments in Constants if necessary op = Operation(opname, [wraparg(arg) for arg in args]) # add it to self, the basic block self.append(op) return op return build # a bunch of operations we support add = opbuilder("add") mul = opbuilder("mul") getarg = opbuilder("getarg") dummy = opbuilder("dummy") lshift = opbuilder("lshift") # some new one for this post alloc = opbuilder("alloc") load = opbuilder("load") store = opbuilder("store") print = opbuilder("print") def bb_to_str(bb: Block, varprefix: str = "var"): def arg_to_str(arg: Value): if isinstance(arg, Constant): return str(arg.value) else: return varnames[arg] varnames = {} res = [] for index, op in enumerate(bb): var = f"{varprefix}{index}" varnames[op] = var arguments = ", ".join( arg_to_str(op.arg(i)) for i in range(len(op.args)) ) strop = f"{var} = {op.name}({arguments})" res.append(strop) return "\n".join(res)
There are two changes to the code from the last post: Operation
instances
have a new .info
field, which is set to None
by default. We will learn
how the info field is used a bit further down. Also, we define some new
operations.
Interpreter
In this post we will mainly concern ourselves with optimizing
programs that allocate memory. We assume that our language is garbage collected
and memory safe. The new operations that we will optimize are alloc
(allocates some new object), store
(stores a value into a fixed field of an
object), load
(loads the value from a field in the object).
We are leaving out a lot of details of a "real" system here, usually an
alloc
operation would get some extra information, for example the type of
the freshly allocated object or at least its size. load
and store
would
typically have some kind of field offset and maybe some information about the
field's type
Here's a simple program that uses these operations:
var0 = getarg(0) obj0 = alloc() store(obj0, 0, var0) var1 = load(obj0, 0) print(var1)
The code allocates a new object obj0
, stores var0
into field 0
of
the object, the loads the same field and prints the result of the load.
Before we get started in writing the optimizer for these operations, let's try
to understand the semantics of the new operations a bit better. To do this, we
can sketch a small interpreter for basic blocks, supporting only getarg
,
alloc
, store
, load
, print
:
def test_interpret(): bb = Block() var0 = bb.getarg(0) obj = bb.alloc() sto = bb.store(obj, 0, var0) var1 = bb.load(obj, 0) bb.print(var1) assert interpret(bb, 17) == 17 class Object: def __init__(self): self.contents: dict[int, Any] = {} def store(self, idx : int, value : Any): self.contents[idx] = value def load(self, idx : int): return self.contents[idx] def get_num(op, index=1): assert isinstance(op.arg(index), Constant) return op.arg(index).value def interpret(bb : Block, *args : tuple[Any]): def argval(op, i): arg = op.arg(i) if isinstance(arg, Constant): return arg.value else: assert isinstance(arg, Operation) return arg.info for index, op in enumerate(bb): if op.name == "getarg": res = args[get_num(op, 0)] elif op.name == "alloc": res = Object() elif op.name == "load": fieldnum = get_num(op) res = argval(op, 0).load(fieldnum) elif op.name == "store": obj = argval(op, 0) fieldnum = get_num(op) fieldvalue = argval(op, 2) obj.store(fieldnum, fieldvalue) # no result, only side effect continue elif op.name == "print": res = argval(op, 0) print(res) return res else: raise NotImplementedError( f"{op.name} not supported") op.info = res
The interpreter walks the operations of a block, executing each one in turn. It
uses the info
field to store the result of each already executed
Operation
. In this interpreter sketch we stop at the first print
that
we execute and return its argument for the simple but bad reason that it makes
test_interpret
easier to write.
Objects in the interpreter are represented using a class Object
, which
stores the object's field into a Python dictionary. As written above, this is a
simplification, in a real system the alloc operation might for example take
some kind of type as an argument, that describes which kinds of fields an
object has and how they are laid out in memory, which would allow more
efficient storage of the content. But we don't want to care about this level of
detail in the post, so using a dict in the interpreter is good enough.
Version 1: Naive Attempt
In many programs, some allocated objects don't live for very long and have a completely predictable lifetime. They get allocated, used for a while, and then there is no way to reference them any more, so the garbage collector will reclaim them. The very first example block had such an allocation:
var0 = getarg(0) obj0 = alloc() store(obj0, 0, var0) var1 = load(obj0, 0) print(var1)
Here obj0
is written to, then read from, and then it's no longer used. We
want to optimize such programs to remove this alloc
operation. The optimized
version of this program would look like this:
var0 = getarg(0) print(var0)
The alloc
, store
and load
operations have been completely removed.
This is a pretty important optimizations for PyPy's JIT: Allocations, memory
reads and writes are quite costly and occur a lot in Python, so getting rid
of as many of them as possible is instrumental for performance.
Implementing the optimization is not a lot of code! However, understanding all the corner cases of the optimization and making sure that the resulting program behave correctly is not completely trivial. Therefore we will develop the optimization step by step, in a test driven fashion: I will start each section with a new test that shows a bug in the version of the optimization that we have so far.
Let's start in a really naive way. Here's the first test we would like to pass, using the example program above:
def test_remove_unused_allocation(): bb = Block() var0 = bb.getarg(0) obj = bb.alloc() sto = bb.store(obj, 0, var0) var1 = bb.load(obj, 0) bb.print(var1) opt_bb = optimize_alloc_removal(bb) # the virtual object looks like this: # obj # ┌──────────┐ # │ 0: var0 │ # └──────────┘ assert bb_to_str(opt_bb, "optvar") == """\ optvar0 = getarg(0) optvar1 = print(optvar0)"""
We will define a class VirtualObject
that is basically identical to
Object
above. But it will not be used by the interpreter, instead we will
use it during optimization.
class VirtualObject: def __init__(self): self.contents: dict[int, Value] = {} def store(self, idx, value): self.contents[idx] = value def load(self, idx): return self.contents[idx]
The structure of the optimizer is going to be like those in the first blog post. The optimizer makes a single pass over all operations. It removes some and emits others.
This first version of the allocation removal optimizer is going to be extremely
optimistic. It simply assumes that all the allocations in the program can be
optimized away. That is not realistic in practice. We will have to
refine this approach later, but it's a good way to start. That means whenever
the optimizer sees an alloc
operation, it removes it and creates a
VirtualObject
object which stores the information that is known during
optimization about the result of the alloc
. Like in the interpreter, the
VirtualObject
is stored in the .info
field of the Operation
instance
that represents the alloc
.
When the optimizer sees a store
operation, it will also remove it and
instead execute the store by calling the VirtualObject.store
method.
Here is one important difference between the interpreter and the optimizer: In
the interpreter, the values that were stored into an Object
(and thus
put into the object's .contents
dictionary) were runtime values, for
example integers or other objects. In the optimizer however, the
fields of the VirtualObject
store Value
instances, either Constant
instances or Operation
instances.
When the optimizer sees a load
operation, it also removes it, and replaces
the load
with the Operation
(or Constant
) that is stored in the
VirtualObject
at that point:
def optimize_alloc_removal(bb): opt_bb = Block() for op in bb: if op.name == "alloc": op.info = VirtualObject() continue if op.name == "load": info = op.arg(0).info field = get_num(op) op.make_equal_to(info.load(field)) continue if op.name == "store": info = op.arg(0).info field = get_num(op) info.store(field, op.arg(2)) continue opt_bb.append(op) return opt_bb
This is the first version of the optimization. It doesn't handle all kinds of difficult cases, and we'll have to do something about its optimism. But, already in this minimalistic form, we can write a slightly more complicated test with two allocations, one object pointing to the other. It works correctly too, both allocations are removed:
def test_remove_two_allocations(): bb = Block() var0 = bb.getarg(0) obj0 = bb.alloc() sto1 = bb.store(obj0, 0, var0) obj1 = bb.alloc() sto2 = bb.store(obj1, 0, obj0) var1 = bb.load(obj1, 0) var2 = bb.load(var1, 0) bb.print(var2) # the virtual objects look like this: # obj0 # ┌──────┐ # │ 0: ╷ │ # └────┼─┘ # │ # ▼ # obj1 # ┌─────────┐ # │ 0: var0 │ # └─────────┘ # therefore # var1 is the same as obj0 # var2 is the same as var0 opt_bb = optimize_alloc_removal(bb) assert bb_to_str(opt_bb, "optvar") == """\ optvar0 = getarg(0) optvar1 = print(optvar0)"""
Version 2: Re-Materializing Allocations
To make it easier to talk about how the optimizer operates, let's introduce
some terminology. As already seen by the choice
of the class name VirtualObject
, we will call an object virtual if the
optimizer has optimized away the alloc
operation that creates the object.
Other objects are equivalently not virtual, for example those that have
existed before we enter the current code block.
The first problem that we need to fix is the assumption that every allocation can be removed. So far we only looked at small programs where every allocation could be removed, or equivalently, where every object is virtual. A program that creates virtual objects, stores into and loads from them, and then forgets the objects. In this simple case removing the allocations is fine. As we saw in the previous section, it's also fine to have a virtual object reference another virtual, both allocations can be removed.
What are the cases were we can't remove an allocation? The first version of the optimizer simply assumed that every allocation can be removed. This can't work. We will replace this assumption with the following simple heuristic:
If a reference to a virtual object a
is stored into an object b
that is not virtual, then a
will also stop being virtual. If an object a
that was virtual stops being virtual, we say that it escapes. ¹
The simplest test case for this happening looks like this:
def test_materialize(): bb = Block() var0 = bb.getarg(0) obj = bb.alloc() sto = bb.store(var0, 0, obj) opt_bb = optimize_alloc_removal(bb) # obj is virtual, without any fields # ┌───────┐ # │ empty │ # └───────┘ # then we store a reference to obj into # field 0 of var0. Since var0 is not virtual, # obj escapes, so we have to put it back # into the optimized basic block assert bb_to_str(opt_bb, "optvar") == """\ optvar0 = getarg(0) optvar1 = alloc() optvar2 = store(optvar0, 0, optvar1)""" # so far, fails like this: # the line: # info.store(field, op.arg(2)) # produces an AttributeError because info # is None
If the optimizer reaches a point where a virtual object escapes (like the
store
operation in the test), the optimizer has already removed the alloc
operation that created the virtual object. If the object escapes, we don't want
to go back in the operations list and re-insert the alloc
operation, that
sounds potentially very complicated. Instead, we re-insert the alloc
operation that will recreate the virtual object at the point of escape using a
helper function materialize
.
def materialize(opt_bb, value: Operation) -> None: assert not isinstance(value, Constant) assert isinstance(value, Operation) info = value.info assert isinstance(info, VirtualObject) assert value.name == "alloc" # put the alloc operation back into the trace opt_bb.append(value)
I've added a number of fairly strong assertions to materialize
to encode our
current assumptions about the situations in which it expects to be called. We
will remove some of them later as we generalize the code.
Now that we have materialize
we need to change optimize_alloc_removal
to
recognize the case of storing a virtual object into a non-virtual one. We can
recognize Operation
instances that produced a virtual object by looking at
their .info
field. If it is None
, the object is not virtual, otherwise
it is. If we store something into a virtual object, we leave the code as above.
If we store a virtual object into an object that is not virtual, we will first
materialize the virtual object, and then emit the store.
def optimize_alloc_removal(bb): opt_bb = Block() for op in bb: if op.name == "alloc": op.info = VirtualObject() continue if op.name == "load": info = op.arg(0).info field = get_num(op) op.make_equal_to(info.load(field)) continue if op.name == "store": info = op.arg(0).info if info: # virtual field = get_num(op) info.store(field, op.arg(2)) continue else: # not virtual # first materialize the # right hand side materialize(opt_bb, op.arg(2)) # then emit the store via # the general path below opt_bb.append(op) return opt_bb
This is the general idea, and it is enough to pass test_materialize
. But of
course there are still a number of further problems that we now need to solve.
Version 3: Don't Materialize Twice
The first problem is the fact that after we materialize a virtual object, it is
no longer virtual. So if it escapes a second time, it should not be
materialized a second time. A test for that case could simply repeat the
store
operation:
def test_dont_materialize_twice(): # obj is again an empty virtual object, # and we store it into var0 *twice*. # this should only materialize it once bb = Block() var0 = bb.getarg(0) obj = bb.alloc() sto0 = bb.store(var0, 0, obj) sto1 = bb.store(var0, 0, obj) opt_bb = optimize_alloc_removal(bb) assert bb_to_str(opt_bb, "optvar") == """\ optvar0 = getarg(0) optvar1 = alloc() optvar2 = store(optvar0, 0, optvar1) optvar3 = store(optvar0, 0, optvar1)""" # fails so far: the operations that we get # at the moment are: # optvar0 = getarg(0) # optvar1 = alloc() # optvar2 = store(optvar0, 0, optvar1) # optvar3 = alloc() # optvar4 = store(optvar0, 0, optvar3) # ie the object is materialized twice, # which is incorrect
We solve the problem by setting the .info
field of an object that we
materialize to None
to mark it as no longer being virtual.
def materialize(opt_bb, value: Operation) -> None: assert not isinstance(value, Constant) assert isinstance(value, Operation) info = value.info if info is None: return # already materialized assert value.name == "alloc" # put the alloc operation back into the trace opt_bb.append(value) # but only once value.info = None # optimize_alloc_removal unchanged
This fixes the problem, only one alloc
is created. This fix also allows
another test case to pass, one where we store a non-virtual into another
non-virtual, code which we cannot optimize at all:
def test_materialize_non_virtuals(): # in this example we store a non-virtual var1 # into another non-virtual var0 # this should just lead to no optimization at # all bb = Block() var0 = bb.getarg(0) var1 = bb.getarg(1) sto = bb.store(var0, 0, var1) opt_bb = optimize_alloc_removal(bb) assert bb_to_str(opt_bb, "optvar") == """\ optvar0 = getarg(0) optvar1 = getarg(1) optvar2 = store(optvar0, 0, optvar1)"""
Version 4: Materialization of Constants
Another straightforward extension is to support materializing constants. A constant is never virtual, so materializing it should do nothing.
def test_materialization_constants(): # in this example we store the constant 17 # into the non-virtual var0 # again, this will not be optimized bb = Block() var0 = bb.getarg(0) sto = bb.store(var0, 0, 17) opt_bb = optimize_alloc_removal(bb) # the previous line fails so far, triggering # the assert: # assert not isinstance(value, Constant) # in materialize assert bb_to_str(opt_bb, "optvar") == """\ optvar0 = getarg(0) optvar1 = store(optvar0, 0, 17)"""
To implement that case, we check for value
being a constant and return
early:
def materialize(opt_bb, value: Operation) -> None: if isinstance(value, Constant): return assert isinstance(value, Operation) info = value.info if info is None: return # already materialized assert value.name == "alloc" # put the alloc operation back into the trace opt_bb.append(value) # but only once value.info = None # optimize_alloc_removal unchanged
Version 5: Materializing Fields
Now we need to solve a more difficult problem. So far, the virtual objects that we have materialized have all been empty, meaning they didn't have any fields written to at the point of materialization. Let's write a test for this:
def test_materialize_fields(): bb = Block() var0 = bb.getarg(0) var1 = bb.getarg(1) obj = bb.alloc() contents0 = bb.store(obj, 0, 8) contents1 = bb.store(obj, 1, var1) sto = bb.store(var0, 0, obj) # the virtual obj looks like this # obj # ┌──────┬──────────┐ # │ 0: 8 │ 1: var1 │ # └──────┴──────────┘ # then it needs to be materialized # this is the first example where a virtual # object that we want to materialize has any # content and is not just an empty object opt_bb = optimize_alloc_removal(bb) assert bb_to_str(opt_bb, "optvar") == """\ optvar0 = getarg(0) optvar1 = getarg(1) optvar2 = alloc() optvar3 = store(optvar2, 0, 8) optvar4 = store(optvar2, 1, optvar1) optvar5 = store(optvar0, 0, optvar2)""" # fails so far! the operations we get # at the moment are: # optvar0 = getarg(0) # optvar1 = getarg(1) # optvar2 = alloc() # optvar3 = store(optvar0, 0, optvar2) # which is wrong, because the store operations # into optvar1 got lost
To fix this problem, we need to re-create a store
operation for every
element of the .contents
dictionary of the virtual object we are
materializing. ²
def materialize(opt_bb, value: Operation) -> None: if isinstance(value, Constant): return assert isinstance(value, Operation) info = value.info if info is None: return # already materialized assert value.name == "alloc" # put the alloc operation back into the trace opt_bb.append(value) # put the content back for idx, val in info.contents.items(): # re-create store operation opt_bb.store(value, idx, val) # only materialize once value.info = None # optimize_alloc_removal unchanged
This is enough to pass the test.
Version 6: Recursive Materialization
In the above example, the fields of the virtual objects contained only constants or non-virtual objects. However, we could have a situation where a whole tree of virtual objects is built, and then the root of the tree escapes. This makes it necessary to escape the whole tree. Let's write a test for a small tree of two virtual objects:
def test_materialize_chained_objects(): bb = Block() var0 = bb.getarg(0) obj0 = bb.alloc() obj1 = bb.alloc() contents = bb.store(obj0, 0, obj1) const = bb.store(obj1, 0, 1337) sto = bb.store(var0, 0, obj0) # obj0 # ┌──────┐ # │ 0: ╷ │ # └────┼─┘ # │ # ▼ # obj1 # ┌─────────┐ # │ 0: 1337 │ # └─────────┘ # now obj0 escapes opt_bb = optimize_alloc_removal(bb) assert bb_to_str(opt_bb, "optvar") == """\ optvar0 = getarg(0) optvar1 = alloc() optvar2 = alloc() optvar3 = store(optvar2, 0, 1337) optvar4 = store(optvar1, 0, optvar2) optvar5 = store(optvar0, 0, optvar1)""" # fails in an annoying way! the resulting # basic block is not in proper SSA form # so printing it fails. The optimized # block would look like this: # optvar0 = getarg(0) # optvar1 = alloc() # optvar3 = store(optvar1, 0, optvar2) # optvar4 = store(optvar0, 0, optvar1) # where optvar2 is an ``alloc`` Operation # that is not itself in the output block
To fix it, materialize
needs to call itself recursively for all the field
values of the virtual object:
def materialize(opt_bb, value: Operation) -> None: if isinstance(value, Constant): return assert isinstance(value, Operation) info = value.info if info is None: return # already materialized assert value.name == "alloc" # put the alloc operation back into the trace opt_bb.append(value) # put the content back for idx, val in sorted(info.contents.items()): # materialize recursively materialize(opt_bb, val) opt_bb.store(value, idx, val) # only materialize once value.info = None # optimize_alloc_removal unchanged
Getting there, the materialization logic is almost done. We need to fix a subtle remaining problem though.
Version 7: Dealing with Object Cycles
The bug we need to fix in this section is a bit tricky, and does not immediately occur in a lot of programs. In fact, in PyPy a variant of it was hiding out in our optimizer until we found it much later (despite us being aware of the general problem and correctly dealing with it in other cases).
The problem is this: a virtual object can (directly or indirectly) point to
itself, and we must carefully deal with that case to avoid infinite recursion in
materialize
. Here's the simplest test:
def test_object_graph_cycles(): bb = Block() var0 = bb.getarg(0) var1 = bb.alloc() var2 = bb.store(var1, 0, var1) var3 = bb.store(var0, 1, var1) # ┌────────┐ # ▼ │ # obj0 │ # ┌──────┐ │ # │ 0: ╷ │ │ # └────┼─┘ │ # │ │ # └─────┘ # obj0 points to itself, and then it is # escaped opt_bb = optimize_alloc_removal(bb) # the previous line fails with an # InfiniteRecursionError # materialize calls itself, infinitely # what we want is instead this output: assert bb_to_str(opt_bb, "optvar") == """\ optvar0 = getarg(0) optvar1 = alloc() optvar2 = store(optvar1, 0, optvar1) optvar3 = store(optvar0, 1, optvar1)"""
The fix is not a big change, but a little bit subtle nevertheless.
We have to change the
order in which things are done in materialize
. Right after emitting the
alloc
, we set the .info
to None
, to mark the object as not virtual.
Only afterwards do we re-create the stores and call materialize
recursively.
If a recursive call reaches the same object, it's already marked as non-virtual,
so materialize
won't recurse further:
def materialize(opt_bb, value: Operation) -> None: if isinstance(value, Constant): return assert isinstance(value, Operation) info = value.info if info is None: return # already materialized assert value.name == "alloc" # put the alloc operation back into the trace opt_bb.append(value) # only materialize once value.info = None # put the content back for idx, val in sorted(info.contents.items()): # materialize recursively materialize(opt_bb, val) opt_bb.store(value, idx, val)
Version 8: Loading from non-virtual objects
Now materialize is done. We need to go back to optimize_alloc_removal
and
improve it further. The last time we changed it, we added a case analysis to the
code dealing with store
, distinguishing between storing to a virtual and to
a non-virtual object. We need to add an equivalent distinction to the load
case, because right now loading from a non-virtual crashes.
def test_load_non_virtual(): bb = Block() var0 = bb.getarg(0) var1 = bb.load(var0, 0) bb.print(var1) # the next line fails in the line # op.make_equal_to(info.load(field)) # because info is None opt_bb = optimize_alloc_removal(bb) assert bb_to_str(opt_bb, "optvar") == """\ optvar0 = getarg(0) optvar1 = load(optvar0, 0) optvar2 = print(optvar1)"""
To fix it, we split the load
code into two cases, leaving the virtual path
as before, and letting the load
from a non-virtual fall through to the
general code at the end of the function.
def optimize_alloc_removal(bb): opt_bb = Block() for op in bb: if op.name == "alloc": op.info = VirtualObject() continue if op.name == "load": info = op.arg(0).info if info: # virtual field = get_num(op) op.make_equal_to(info.load(field)) continue # otherwise not virtual, use the # general path below if op.name == "store": info = op.arg(0).info if info: # virtual field = get_num(op) info.store(field, op.arg(2)) continue else: # not virtual # first materialize the # right hand side materialize(opt_bb, op.arg(2)) # then emit the store via # the general path below opt_bb.append(op) return opt_bb
Version 9 (Final): Materialize on Other Operations
We're almost at the end now. There's one final generalization left to do. We
started with the heuristic that storing a virtual into a non-virtual would
escape it. This should be generalized. Every time we pass a virtual into any
operation where it is not the first argument of a load
and a store
should also escape it (imagine passing the virtual to some function call).
Let's test this as usual with our print
operation:
def test_materialize_on_other_ops(): # materialize not just on store bb = Block() var0 = bb.getarg(0) var1 = bb.alloc() var2 = bb.print(var1) opt_bb = optimize_alloc_removal(bb) assert bb_to_str(opt_bb, "optvar") == """\ optvar0 = getarg(0) optvar1 = alloc() optvar2 = print(optvar1)""" # again, the resulting basic block is not in # valid SSA form
To fix this, we will take the call to materialize
out of the store
code
path and instead put it into the generic code path the end of the while
loop:
# materialize is unchanged def materialize(opt_bb, value: Value) -> None: if isinstance(value, Constant): return assert isinstance(value, Operation) info = value.info if not info: # Already materialized return assert value.name == "alloc" opt_bb.append(value) value.info = None for idx, val in sorted(info.contents.items()): materialize(opt_bb, val) opt_bb.store(value, idx, val) def optimize_alloc_removal(bb): opt_bb = Block() for op in bb: if op.name == "alloc": op.info = VirtualObject() continue if op.name == "load": info = op.arg(0).info if info: # virtual field = get_num(op) op.make_equal_to(info.load(field)) continue if op.name == "store": info = op.arg(0).info if info: # virtual field = get_num(op) info.store(field, op.arg(2)) continue # materialize all the arguments of # operations that are put into the # output basic block for arg in op.args: materialize(opt_bb, arg.find()) opt_bb.append(op) return opt_bb
That's it, we're done. It's not a lot of code, but actually quite a powerful optimization. In addition to removing allocations for objects that are only used briefly and in predictable ways, it also has another effect. If an object is allocated, used in a number of operations and then escapes further down in the block, the operations in between can often be optimized away. This is demonstrated by the next test (which already passes):
def test_sink_allocations(): bb = Block() var0 = bb.getarg(0) var1 = bb.alloc() var2 = bb.store(var1, 0, 123) var3 = bb.store(var1, 1, 456) var4 = bb.load(var1, 0) var5 = bb.load(var1, 1) var6 = bb.add(var4, var5) var7 = bb.store(var1, 0, var6) var8 = bb.store(var0, 1, var1) opt_bb = optimize_alloc_removal(bb) assert bb_to_str(opt_bb, "optvar") == """\ optvar0 = getarg(0) optvar1 = add(123, 456) optvar2 = alloc() optvar3 = store(optvar2, 0, optvar1) optvar4 = store(optvar2, 1, 456) optvar5 = store(optvar0, 1, optvar2)"""
Note that the addition is not optimized away, because the code from this blog post does not contain constant folding and the other optimizations from the last one. Combining them would not be too hard though.
Conclusion
That's it! The core idea of PyPy's allocation removal optimization in one or two screens of code. The real implementation has a number of refinements, but the core ideas are all here.
I'm not going to show any benchmark numbers or anything like that here, if you are interested in numbers you could look at the evaluation Section 6. "Implementation and Evaluation" of the paper that describes the work.
There's a complementary optimization that improves load
and store
operations for objects that are not virtual. I'll probably not write that
down as another post, but Max Bernstein and I developed that together on a
PyPy Twitch channel channel a few weeks ago, here's the recording:
Footnotes
¹ This is how PyPy uses the terminology, not really used consistently by other projects. The term "escape" is fairly standard throughout the escape analysis literature. The term "virtual" was used originally in Armin Rigo's Psyco but is e.g. also used by the paper Partial Escape Analysis and Scalar Replacement for Java.
² The order in which we put the store operations back is relying on dictionary iteration order, which is insertion order. That's not a bad ordering, we could also be explicit and sort the fields in some order (ideally the order in which the object lays them out in memory).
Düsseldorf HPy/PyPy/GraalPy sprint September 19-23rd 2022
The programming language group of the Computer Science department of Heinrich-Heine Universität Düsseldorf is happy to invite everybody to another sprint in Düsseldorf, from the 19th to the 23rd of September 2022. This is a fully public sprint, everyone and particularly newcomers are welcome to join us! The goal is to bring together people from the HPy, PyPy, GraalPy and CPython communities.
Topics and goals
work on HPy APIs, discussions around next steps for the project
continuing new and ongoing ports to HPy, including Cython, NumPy, Pillow, Matplotlib
3.10 support on PyPy and GraalPy
preparing the next PyPy release
discussions around ways to improve collaboration between the different Python implementations
What is a sprint?
The experience of the PyPy project has shown the benefits of regular sprints. They are focussed one week physical meetings where people pair-program on new features and discuss future plans. Coming to one is a great way to get started with a project!
Location
The sprint will take place in a seminar room of the computer science department. It is in the building 25.12, room 02.50 (second floor) of the university campus. For travel instructions see
We ask participants to wear masks during the indoor working hours.

Wiegels, CC BY 3.0, via Wikimedia Commons
Exact times
Work days: starting September 19th (~morning), ending September 23rd (~afternoon). We will do a to-be-planned social activity on Wednesday afternoon.
Registration
Please register by editing this file or by opening a pull request:
https://foss.heptapod.net/pypy/extradoc/-/blob/branch/extradoc/sprintinfo/ddorf2022/people.txt
or by sending a quick mail to the pypy-dev mailing list: